This article presents a comparative study of two advanced generative AI models, Stability AI 3 (SD3) and DALL·E 3. Both models represent significant advancements in the field of AI-driven image generation, each with unique features and capabilities. We will evaluate their architectures, performance, training datasets, output quality, and potential applications. Additionally, the paper will explore the ethical considerations and limitations associated with each model.
Introduction
Generative AI models have evolved rapidly, with Stability AI 3 (SD3) and DALL·E 3 standing out as two of the most sophisticated systems in 2024. Stability AI 3, developed by Stability AI, and DALL·E 3, developed by OpenAI, have pushed the boundaries of image generation, enabling the creation of highly detailed and imaginative visuals from textual descriptions. This study aims to compare these models to understand their strengths, weaknesses, and potential use cases.
Architectural Overview
Stability AI 3 (SD3)
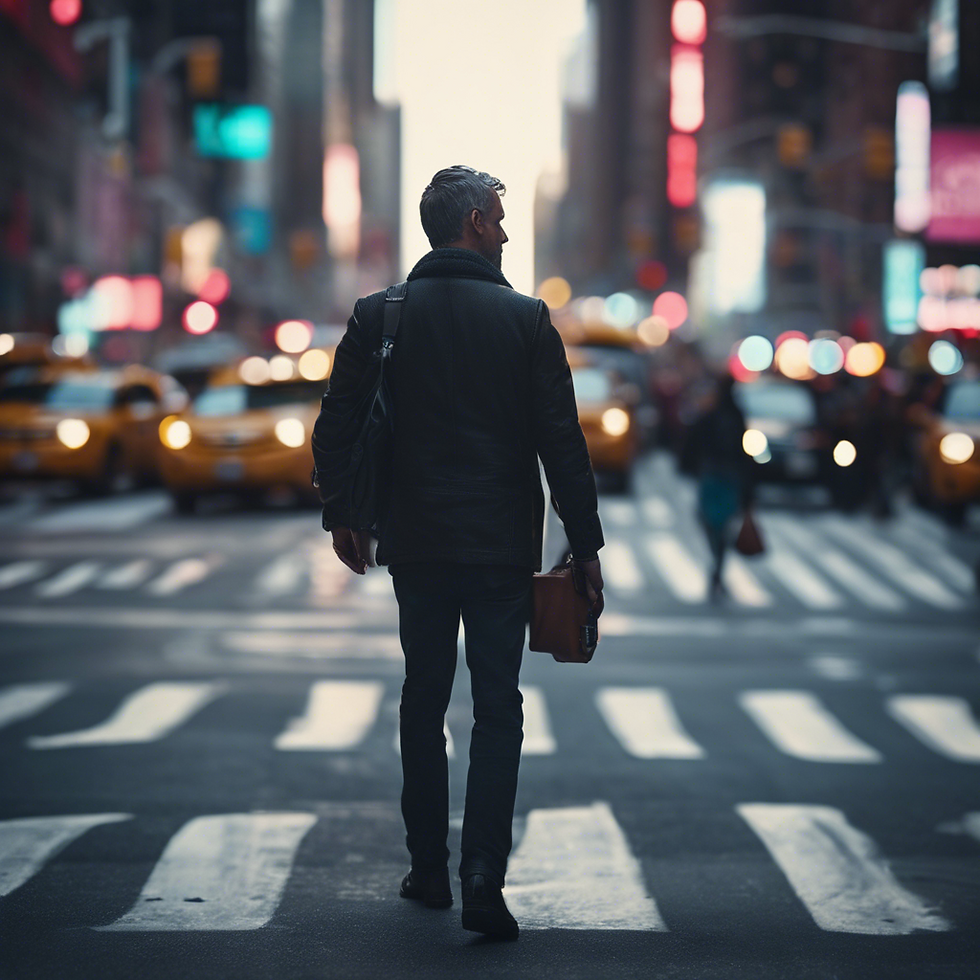
Stability AI 3 utilizes a novel architecture combining elements of diffusion models and transformer networks. This hybrid approach allows SD3 to generate images with high fidelity and diversity. The model leverages a diffusion process to iteratively refine noise into coherent images, guided by a transformer that interprets and conditions the input text.
DALL·E 3
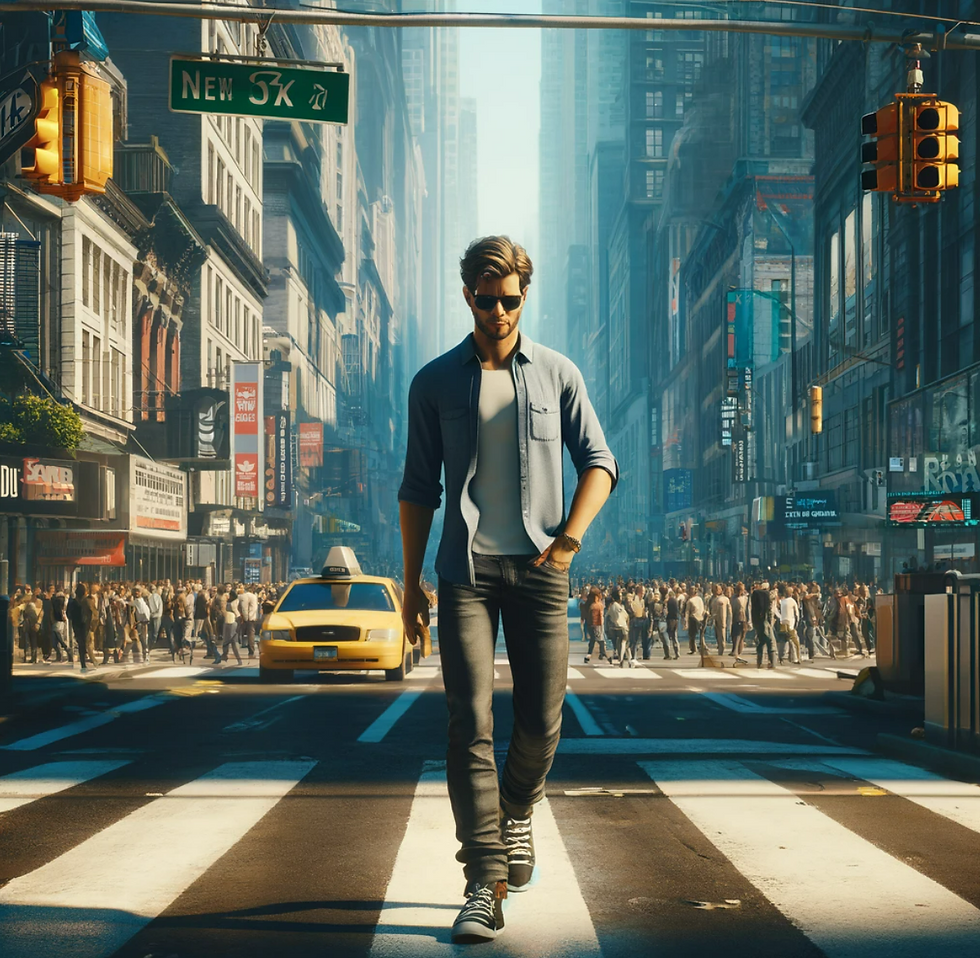
DALL·E 3 builds upon the foundation of its predecessors, using a transformer-based architecture designed specifically for text-to-image generation. It employs a large-scale autoregressive model that predicts the next pixel based on the input text and previously generated pixels. This iterative process results in detailed and contextually accurate images.
Training Datasets
The quality and diversity of training data play crucial roles in the performance of generative models.
Stability AI 3
SD3 was trained on a diverse dataset comprising millions of images and their corresponding textual descriptions. This dataset includes a wide range of categories, from natural landscapes to abstract concepts, ensuring the model's versatility. The data was curated to minimize biases and promote ethical use.
DALL·E 3
DALL·E 3 was trained on an even larger and more diverse dataset, incorporating images from various sources, including licensed, publicly available, and proprietary datasets. OpenAI placed a strong emphasis on data quality and ethical considerations, filtering out harmful and biased content to create a more inclusive model.
Performance Evaluation
Performance metrics for generative models include fidelity, diversity, coherence, and user satisfaction.
Stability AI 3
SD3 excels in generating highly detailed and realistic images. Its hybrid architecture allows for intricate textures and complex scenes. User studies indicate high satisfaction with the model's ability to interpret and visualize abstract concepts accurately. However, some limitations include occasional overfitting to common themes and a tendency to struggle with extremely complex or novel prompts.
DALL·E 3
DALL·E 3 is renowned for its creativity and ability to produce imaginative and novel images. The autoregressive model excels at maintaining coherence across the entire image, resulting in visually pleasing outputs. Users appreciate its versatility and the richness of generated images, though it occasionally produces less realistic results compared to SD3.
Applications
Both models have a wide range of applications, from creative industries to practical use cases.
Stability AI 3
SD3 is particularly well-suited for applications requiring high realism, such as virtual reality, video game design, and digital art. Its ability to generate detailed textures and lifelike scenes makes it a valuable tool for professionals in these fields.
DALL·E 3
DALL·E 3's strength lies in its creativity and versatility, making it ideal for advertising, conceptual art, and educational content. Its ability to generate unique and imaginative visuals opens up new possibilities for creative professionals and educators.
Ethical Considerations
Both Stability AI 3 and DALL·E 3 developers have implemented measures to address ethical concerns.
Stability AI 3
Stability AI has focused on minimizing biases in the training data and implementing safeguards to prevent the generation of harmful or inappropriate content. Additionally, they have emphasized transparency in the model's limitations and potential misuse.
DALL·E 3
OpenAI has prioritized ethical considerations by filtering training data and employing robust monitoring mechanisms to detect and mitigate harmful outputs. They also promote responsible use by providing guidelines and tools to ensure the model is used ethically.
Limitations
Despite their advancements, both models have limitations.
Stability AI 3
SD3 may occasionally produce less creative or novel images compared to DALL·E 3. It also requires substantial computational resources, making it less accessible for smaller organizations or individual users.
DALL·E 3
DALL·E 3, while highly creative, sometimes sacrifices realism for novelty. It can also generate less accurate interpretations of complex or ambiguous prompts. The model's large size and computational demands are additional challenges.
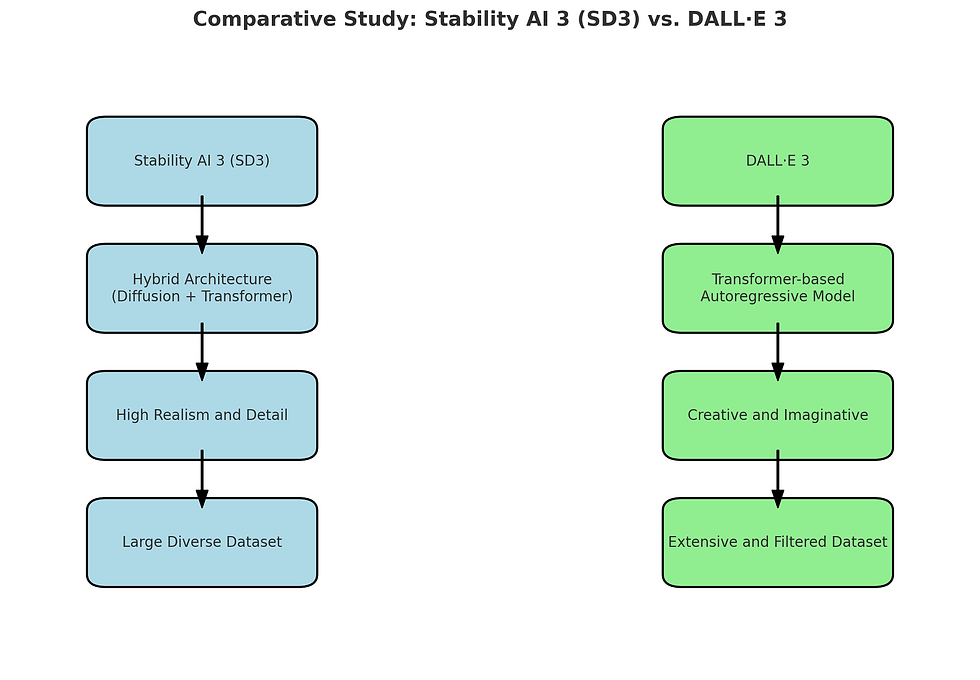
Conclusion
Stability AI 3 (SD3) and DALL·E 3 represent significant strides in generative AI, each excelling in different areas. SD3 is ideal for applications demanding high realism and detail, while DALL·E 3 is better suited for creative and imaginative tasks. Both models have addressed ethical considerations, but ongoing efforts are needed to refine their capabilities and mitigate limitations. Future research should focus on improving accessibility, reducing biases, and exploring new applications for these powerful tools.
References
Stability AI. (2024). Stability AI 3: Architecture and Applications. [Stability AI website].
OpenAI. (2024). DALL·E 3: A Creative AI for the Future. [OpenAI website].
Brown, T. B., et al. (2020). Language Models are Few-Shot Learners. [ArXiv].
Ho, J., et al. (2020). Denoising Diffusion Probabilistic Models. [ArXiv].
Comentários